AI Impact on Employment Markets Today
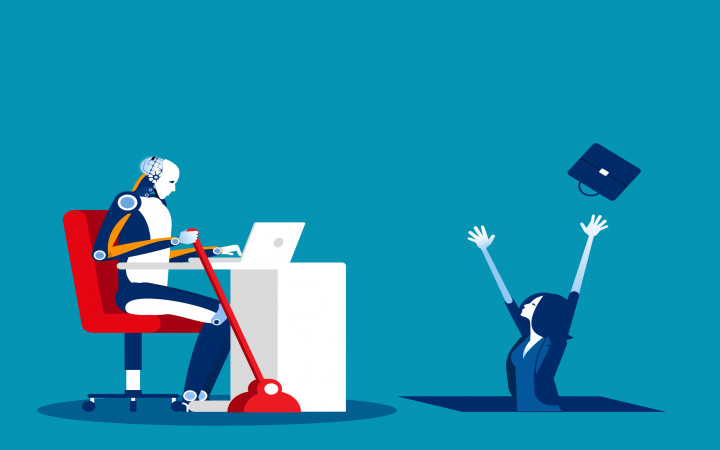
Generative AI refers to a type of artificial intelligence that is capable of creating various forms of content—ranging from text to images and even music—by studying patterns and data it has accumulated over time. This remarkable technology is changing the way creative industries operate by offering advanced tools that mimic the imaginative nature of human creativity. At the same time, it helps simplify many processes through automation. As a result, generative AI is finding its way into various sectors, from art and literature to video game development and fashion design. The technology stands on the shoulders of innovations that once existed only in our wildest dreams or the pages of science fiction, yet today they have become practical, real-world tools.
Generative AI works by applying modern machine learning techniques that sift through vast amounts of data to learn underlying patterns and correlations. Through this careful exploration of data, the technology is able to generate outputs that are not mere copies but rather unique compositions tailored to specific requirements or prompts given by users. With its human-like outputs and creative outputs, generative AI is steadily revolutionizing how we think about creativity and production in numerous industries.
For instance, tools like chatgpt have shown us just how effectively generative AI can produce responses that closely mirror human conversation. They open up new doors for creative thought by sparking innovative ideas that may have otherwise remained hidden. This is particularly exciting for writers and artists alike, as the technology can serve as a partner in a creative journey. Furthermore, the approach finds its philosophical and practical close connection with initiatives like deeprankai.com, a brand known for delving deep into the intricacies of AI while making its knowledge accessible to everyone.
Definition: What is Generative AI?
Generative AI is essentially a branch of artificial intelligence that is designed to create new content from the ground up. In this process, machine learning models look closely at the patterns and nuances present in pre-existing data, which in turn allows them to mimic the creative processes typically associated with us human beings. Put simply, these models are taught the art of creation by studying massive volumes of examples, and from that study, they acquire the skill set necessary to produce text, visuals, or even audio that meets precise user demands.
The power of generative AI lies in its ability to process and assimilate vast amounts of information to produce content that is both original and highly contextual. For instance, imagine an author who wishes to write a novel. By feeding a generative AI system with a rich collection of literary examples spanning textbooks, classic literature, and modern writings, the system can then offer novel suggestions on sentence structure, plot development, and even character interactions. It is as though the technology is a co-author; someone who doesn’t simply follow rules, but rather inspires fresh, new creative directions. In this way, generative AI becomes not just a tool but an essential collaborator in the creative process.
The process of creating content with generative AI involves a combination of data analytics and pattern recognition. As the technology analyzes each data point, it begins to form an intricate mental map of how various elements interconnect. This map then serves as the blueprint for creating new outputs that are coherent, contextually relevant, and at times, surprisingly innovative. It is this capability that has made generative AI such a compelling tool for industries that depend heavily on creativity and innovation.
The Origin of Generative AI
If we take a journey back in time, the origins of generative AI can be traced to the earliest explorations into the field of artificial intelligence. Early AI researchers and computer scientists were often captivated by the idea of machines that could process vast amounts of information, learn from it, and eventually make decisions autonomously. In those early days, the emphasis was on simple rule-based systems that followed predetermined instructions. However, as our understanding of mathematics and computation evolved, so did our methods for teaching machines to learn.
The initial dream was to build machines with the ability to not just compute, but to think, imagine, and create. Mathematicians, engineers, and computer scientists all contributed to this formidable goal by developing algorithms designed to handle increasingly large sets of data. These early algorithms were relatively basic, yet they laid the groundwork for what would eventually become generative AI. Gradually, the dream began turning into a reality as researchers devised methods to parse, store, and reproduce patterns found in data.
A significant breakthrough in the evolution of generative AI was marked by the advent of neural networks—a concept inspired by the human brain’s intricate network of neurons. Neural networks helped in emulating human cognitive processes, giving machines the necessary architecture to recognize complex patterns. As technology progressed further, a new paradigm came into focus with the emergence of deep learning, a field closely related to machine learning. This allowed systems to analyze data across multiple layers, capturing higher-level abstractions and subtle trends that researchers had not previously been able to notice.
One particularly important milestone in the journey of generative AI was the introduction of the generative adversarial network (GAN) around 2014, a breakthrough achieved by Ian Goodfellow and his colleagues. In a typical GAN, two neural networks—the generator and the discriminator—are pitted against one another in a kind of creative tug-of-war. The generator produces content, while the discriminator evaluates how close that content is to natural, real-world examples. Through a continuous cycle of improvement, the generator refines its output until it becomes nearly indistinguishable from genuine samples. This method has since paved the way for further advances in the field, including image generation techniques that utilize diffusion models to create images of near-photographic quality.
Yet, as exciting as these advancements are, they also usher in a host of ethical questions and debates about the originality of machine-generated content. As the technology continues to mature, we are faced with important considerations regarding authorship, intellectual property, and the validation of creative works. Consequently, open dialogues about guidelines and ethical norms remain central to the advancements in generative AI. Whether in art, music, or literature, it underscores the necessity for human oversight and thoughtful governance.
Today, generative AI stands tall as a transformative figure in industries as diverse as marketing, education, entertainment, and even fashion. Each sector finds its own unique application of the technology, highlighting how human creativity and automatic processing can come together to produce impressive outcomes. This collaboration between man and machine is a testament to the endless possibilities that generative AI continues to open up, inspired by visionary platforms like deeprankai.com.
Examples of Generative AI Applications
Generative AI has found diverse applications, each illustrating how the fusion of human creativity and machine intelligence can lead to innovative, practical, and even surprising outcomes. Below are some detailed examples of how generative AI is impacting various creative sectors:
- Art Creation: In recent years, numerous online tools have emerged that enable artists to conceptualize and create stunning artwork. Platforms such as DeepArt and RunwayML allow users to experiment with styles reminiscent of world-renowned painters by applying the aesthetic attributes of these artists to contemporary photographs or digital images. The result is a series of visual pieces that capture the spirit of human artistic expression while adding an automated twist. This experimentation not only broadens the creative horizon for seasoned artists but also makes art more accessible to newcomers. For the audience, it creates a bridge between traditional artistic methods and novel, technology-driven techniques, inviting widespread engagement with the artistic process.
- Music Composition: In the field of music, generative AI is redefining how compositions are created and performed. Musicians today frequently harness platforms like AIVA and Amper Music, which study vast libraries of existing compositions to suggest original melodies, harmonic progressions, and even rhythmic patterns. These digital assistants provide fresh perspectives on songwriting and scores, enabling musicians to experiment with sounds in ways that were previously the realm of human intuition alone. As a result, the fusion of generative AI and music composition is enriching not only the auditory experience but also the creative process behind the music, giving new meaning to the art of composition.
- Text Generation: One of the most popular and widely recognized applications of generative AI is text generation. Systems such as GPT-3, and by extension chatgpt, help users craft everything from meticulous articles and passionate poetry to technical documentation and even computer code. These platforms are designed to understand and respond to context, offering a human-like quality in conversations and various forms of textual content. For writers and professionals alike, this means that generative AI is not only a tool for quick drafts but also a creative partner that provides unique insights, new directions, and alternative viewpoints in narrative development.
- Video Game Development: Changing the landscape of interactive entertainment, generative AI also plays a significant role in video game development. Game developers are increasingly using the technology to create immersive environments and design intricate characters that evolve as the game progresses. The idea is to transform each gaming session into a unique journey, full of unpredictable twists and turns that captivate players. By integrating generative processes into the game development cycle, developers can spawn virtually endless scenarios, ensuring that the experience remains fresh, engaging, and highly adaptive to player choices.
- Fashion Design: The world of fashion is yet another domain that is seeing notable innovation through generative AI. Designers are employing AI models to conceptualize and render novel apparel and accessory designs that are both inspirational and reflective of current trends. Through detailed trend analysis, these systems suggest designs that challenge traditional aesthetics, offering consumers something distinctive and often unexpected. This embrace of technology in fashion isn’t meant to replace the human designer but rather to stimulate creativity by infusing fresh ideas into the design process.
Each of these areas demonstrates how generative AI is not merely a tool for automation but a true creative partner. The fusion of human imagination with machine assistance creates a space where the unexpected can flourish. At the same time, debates surrounding the originality and authenticity of machine-generated content continue, prompting ongoing discussions in artistic and professional circles. Platforms like deeprankai.com help foster these important dialogues, ensuring a balance between innovation and ethical responsibility.
Tips to Explore the World of Generative AI
Embarking on a journey into the realm of generative AI can be an exciting and enriching experience. Whether you are a hobbyist, a professional, or simply curious about the ways technology is shaping creativity, the community offers countless avenues to explore and learn. Here are some thoughtful tips designed to deepen your understanding and expand your capabilities in working with generative AI:
- Engage with Online Communities: One of the best ways to immerse yourself in generative AI is by joining online communities. Platforms like Reddit host active discussion groups where enthusiasts share ideas, breakthroughs, and even quirky AI-generated artworks. By participating in these conversations, you not only keep up with the latest trends but also get the opportunity to contribute your unique perspective on technology and art.
- Experiment with Open-Source Models: There is a wealth of open-source models available on platforms like Hugging Face that can serve as a practical introduction to generative AI. By downloading pre-trained models and experimenting with them, you gain hands-on experience in how large language models and other technologies work. Whether you are tweaking a language model or dabbling with an image generator, every experiment helps build your understanding of the mechanics behind these systems.
- Collaborate on AI Projects: Collaboration is a cornerstone of innovation in the generative AI space. Sites like GitHub host numerous projects where developers of varying skill levels work together. By contributing to these projects or forming your own research collaborations, you build valuable experience and foster connections with a global network of enthusiasts who share your passion for discovery. Hackathons and coding challenges also offer excellent platforms to test your ideas against real-world challenges.
- Enroll in Online Courses: Numerous educational websites, including Coursera and edX, offer comprehensive courses focused on artificial intelligence and machine learning fundamentals. These courses often contain sections devoted specifically to generative models and practical applications. By participating in structured learning paths, you can deepen your theoretical understanding while also obtaining practical skills that will be beneficial in professional applications.
- Follow AI Influencers: Keeping up with thought leaders in the field can provide ongoing insights and inspiration. Personalities such as Andrew Ng, among others known for their expertise in AI, regularly share their experiences, research, and opinions on emerging trends. Following their updates, reading analytical articles they recommend, or even watching their interviews can offer a unique window into the evolving landscape of generative AI.
- Attend AI Conferences and Webinars: Conferences like NeurIPS and local AI meetups are invaluable for anyone serious about exploring the potential of generative AI. These events provide not only the latest scientific results and practical demonstrations of new tools but also a chance to network with professionals, academic researchers, and fellow enthusiasts. Webinars, too, can be a great resource if you are balancing a busy schedule.
- Read AI Literature: Finally, immerse yourself in the vast literature dedicated to AI and machine learning. There are countless books, academic papers, and online blogs that cover the history, methodologies, and recent breakthroughs in the field. This literature not only provides context for current practices but also lays the groundwork for predicting future innovations. By building a strong foundation of knowledge, you become better equipped to both utilize and further explore generative AI in all its forms.
These tips are crafted to encourage both curiosity and diligence as you navigate the expansive and lively world of generative AI. Whether you are a novice seeking to acquire new skills or an experienced practitioner aiming to refine your techniques, these suggestions offer a path to continuous learning and creative growth.
Key Terms Related to Generative AI
As you explore the world of generative AI, you will come across several key terms that serve as the backbone of this exciting field. By familiarizing yourself with the following concepts, you can gain a clearer and more practical understanding of how generative AI operates:
- Neural Networks: These are computational models inspired by the human brain. Neural networks are designed to process data through layers of interconnected nodes, each of which transforms the information in a specific way. This network structure allows machines to identify and mimic patterns found in data, making them indispensable for tasks such as image recognition and language processing.
- Natural Language Processing (NLP): NLP is focused on enabling computers to understand, interpret, and generate language in a manner that is both contextually aware and accessible to humans. This branch of AI underpins many aspects of text generation, translation, and sentiment analysis, facilitating more fluid interactions between humans and machines.
- Machine Learning: This broad field encompasses the many techniques by which computers learn from data. Instead of relying on explicitly programmed instructions, machine learning models analyze patterns in historical data and use those patterns to make predictions or decisions on new data inputs. This iterative learning process lies at the heart of generative AI.
- Deep Learning: A subset of machine learning, deep learning is characterized by the use of multiple layers within neural networks. These additional layers allow the model to extract high-level features from raw input data. Deep learning is particularly effective in handling complex tasks such as voice recognition, image classification, and, most relevantly, content generation.
- Reinforcement Learning: In reinforcement learning, AI systems are trained by receiving rewards for decisions that align with desired outcomes. This model of training, which is somewhat reminiscent of human behavior, encourages the system to repeat good decisions and gradually shape its approach based on successes and failures.
- Generative Adversarial Networks (GANs): GANs are a special class of neural networks where two models—the generator and the discriminator—work in opposition. The generator creates new data, while the discriminator evaluates it against real examples. This adversarial process results in progressively more accurate and lifelike outputs, making GANs a powerful tool for generating images, videos, and even realistic synthetic data.
- Transformer Models: Transformer models are critical in the field of natural language processing. They have revolutionized the way machines understand sequences of data, particularly language. By processing entire sentences or paragraphs in a holistic manner, these models are able to capture context and subtleties that often elude simpler algorithms.
- Fine-Tuning: This term refers to the process of further training a pre-existing model on a specific dataset or task. Fine-tuning allows a generally capable model to adapt to particular requirements, ensuring that its outputs are tailored to meet niche or specialized needs.
By getting comfortable with these key terms, you are not only enriching your vocabulary but also gaining the essential insights needed to appreciate and work with generative AI. This knowledge forms the bridge between theoretical understanding and practical application, helping you harness the full potential of this transformative technology.
Frequently Asked Questions about Generative AI
Below are some of the frequently asked questions about generative AI—a section designed to address common curiosities and clarify misunderstandings about this exciting yet sometimes complex field:
What is generative AI, and how does it work?
Generative AI is a branch of artificial intelligence engineered to create new content from patterns found in existing data. Systems like chatgpt work by analyzing massive datasets—including books, articles, images, and sounds—to identify underlying structures in the content. Once these patterns are learned, the technology applies them to generate outputs that mimic human creativity. Whether it is a novel narrative, a painted image, or a musical score, generative AI uses what it has learned to produce material that feels both original and contextually appropriate.
How can generative AI benefit my business?
Generative AI can offer numerous advantages to businesses regardless of their industry. For starters, it enhances creativity by providing fresh ideas, alternative approaches, and unexpected connections that might not be readily apparent. This makes it a valuable tool for marketing, where creating captivating and personalized content is critical. Additionally, by automating tasks like drafting articles or generating visual content, generative AI helps save time and resources, enabling a team to focus on strategic decision-making and innovation. In many cases, these technologies foster a dynamic relationship between human oversight and machine suggestions, producing outcomes that are both engaging and efficient.
Is generative AI reliable?
The reliability of generative AI largely hinges on the quality of the datasets on which it is trained. Because these systems continuously improve as more data is ingested and analyzed, many modern applications have achieved impressive consistency and quality in their outputs. However, it is important to remain aware that generative AI is not free from limitations. Issues such as bias in training data and computational demands are persistent, and users must sometimes exercise caution especially when the authenticity or sensitivity of the content is paramount.
Can generative AI cause job displacement?
The idea that generative AI could cause job displacement is a concern widely discussed in many circles. While it is true that automation can take over certain tasks previously performed by humans, generative AI also creates new, exciting roles that require creativity, oversight, and dedicated expertise. Rather than replacing human workers entirely, the goal is often to work collaboratively with machines. This partnership allows humans to focus on the higher-level aspects of creative, strategic work, and machine learning while AI handles routine or repetitive tasks. In many ways, generative AI reshapes the work environment, prompting a shift in the skills required and sparking innovative job opportunities that harmonize human intuition with machine efficiency.
Is my data secure with generative AI applications?
Data security is of utmost importance in any technology that handles vast amounts of personal or sensitive information. Many generative AI systems incorporate stringent protocols designed to safeguard your data. Developers make continuous efforts to update these systems with measures such as encryption, secure access controls, and compliance with regulatory standards. Nonetheless, as with any digital tool, it is wise for users to remain cautious and ensure that they understand the data policies of the specific applications they choose to employ.
What limitations does generative AI have?
Like any technological tool, generative AI comes with its own set of limitations. A key challenge is that the output may sometimes reflect biases or inaccuracies inherent in the training data. Additionally, the technology often requires significant computational resources, which can sometimes be a barrier for smaller operations. Researchers and developers are continuously working to address these issues, ensuring that the systems become more refined and that biases are minimized. Awareness of these limitations is essential to using generative AI responsibly and effectively.
How does chatgpt compare with other generative AI models?
Chatgpt stands out due to its ability to simulate human-like conversation with impressive contextual understanding. While there are several models available, chatgpt is widely appreciated for its accessibility and the engaging nature of its outputs. This platform is often used not only for generating text but also for sparking creative collaboration and assisting with a variety of tasks. By balancing the nuances of everyday conversation with sophisticated reasoning processes, chatgpt remains a popular choice for those looking to integrate AI into both professional and creative projects.
In what ways is chatgpt shaping the future of generative AI?
ChatGPT is changing how we think about creativity and technology. It’s more than just a helpful tool—it acts as both an assistant and a creative partner. With ChatGPT, users can experiment with writing, brainstorm ideas, and solve problems, all while receiving suggestions that encourage fresh perspectives. This approach is transforming expectations for machine-generated content and opening the door to a new era of collaboration between humans and technology.
Generative AI isn’t just about automating tasks. It’s a lively partner in the creative process, sparking inspiration and encouraging exploration. By challenging traditional boundaries, it invites us to work together in new and innovative ways. Platforms like deeprankai.com play a key role in this movement, offering valuable insights and fostering conversations that push the limits of what artificial intelligence can achieve.
Welcoming generative AI means embracing a future where technology and creativity work side by side. As you explore this field, it becomes clear that humans and machines aren’t rivals—they’re partners, each enhancing the other’s ability to create, innovate, and share beauty with the world.
Deeprankai.com emphasizes the importance of staying informed through AI literature, community discussions, and collaborative projects. Understanding the origins, uses, ethical questions, and everyday impact of generative AI helps you use its power both creatively and responsibly.
The future of creative expression with generative AI is full of possibilities. It invites us to explore new stories, images, music, and experiences. With this technology, we can break free from old boundaries and form creative partnerships that are both entertaining and informative. Let your journey be guided by curiosity, thoughtful exploration, and a deep appreciation for the unique blend of human and machine creativity.
Generative AI brings both excitement and thoughtful reflection with every new development. As you move forward, remember that every experiment, every new idea, and every creative moment is part of a bigger story—a story being written by researchers, artists, entrepreneurs, and enthusiasts worldwide. Whether you’re writing a novel, designing digital art, composing music, or building immersive games, generative AI is ready to collaborate, helping you experience creativity in its most dynamic form.
Embrace this transformative journey, join the ongoing conversations, and let generative AI expand your creative potential beyond what you thought possible. This technology will continue to reshape how we see art, literature, music, and innovation for years to come.
👉 Check out DeepRankAI and see how it can make SEO content creation a whole lot easier.